Imagine you’re a marketing manager trying to understand the factors influencing your product sales. You gather data on price, advertising spend, seasonality, and competitor activity. But how do you sift through this information to identify the most impactful drivers? This is where applied regression analysis and other multivariable methods come into play, providing powerful tools for unraveling complex relationships within data.
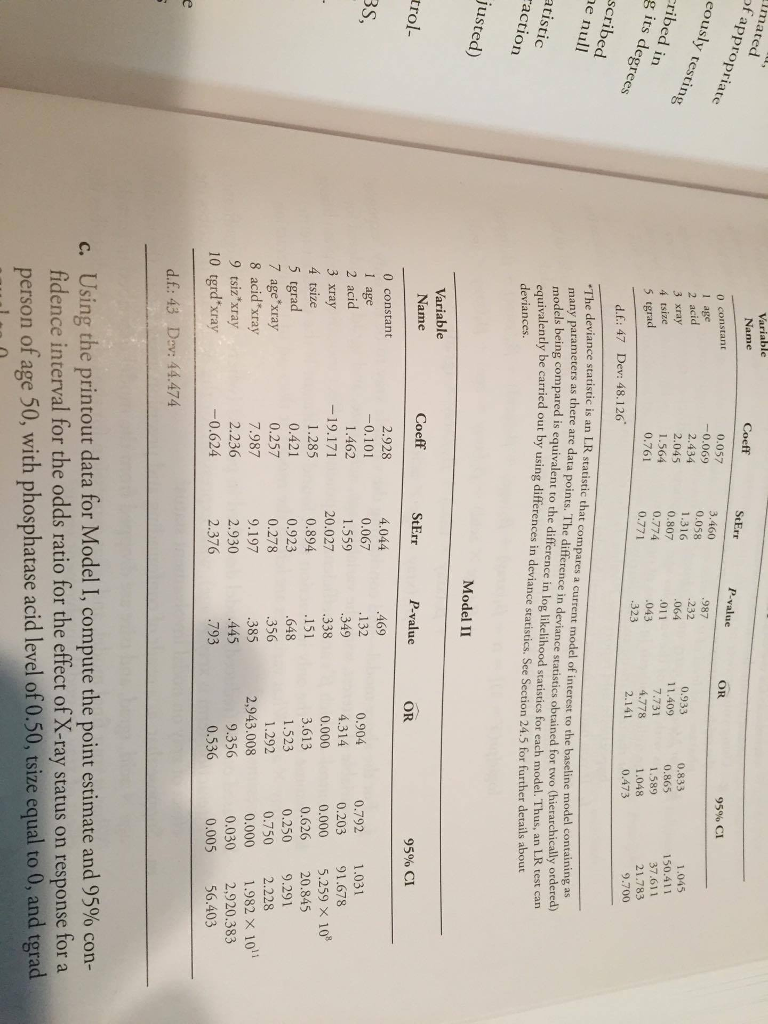
Image: www.chegg.com
These techniques are not just for marketing professionals; they are essential for researchers, scientists, and anyone seeking to make data-driven decisions. From predicting financial markets to understanding climate change, applied regression analysis and similar methods offer deep insights that can shape the future.
Demystifying Multivariable Methods
Understanding the Basics
At its core, applied regression analysis involves modeling the relationship between a dependent variable (the outcome you want to predict) and several independent variables (factors that influence the outcome). This involves fitting a mathematical equation that best represents the data, revealing how changes in independent variables affect the dependent variable.
Multivariable methods encompass a wider family of statistical techniques, including:
- Multiple Linear Regression: Predicting a continuous dependent variable (like sales) from several independent variables.
- Logistic Regression: Determining the probability of a binary outcome (like customer churn) based on multiple predictors.
- Analysis of Variance (ANOVA): Testing differences between group means based on multiple factors.
- Principal Component Analysis (PCA): Reducing the dimensionality of complex datasets by identifying underlying patterns.
Beyond Simple Correlations
The beauty of multivariable methods lies in their ability to analyze complex relationships. While simple correlations can show associations, they can’t tell us causation. Regression analysis helps us isolate the impact of each independent variable, considering all other factors simultaneously. For example, we might discover that while advertising spend is generally positively correlated with sales, its impact is significantly reduced when a competitor launches a similar product.
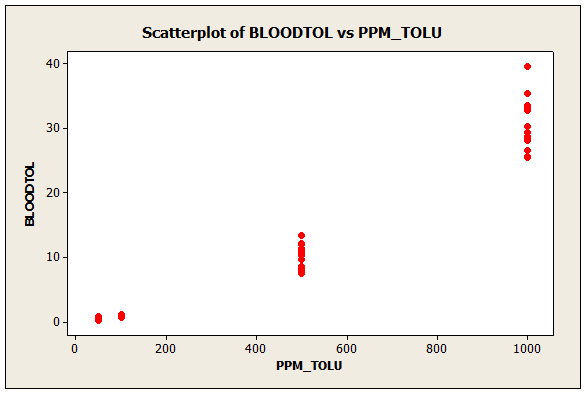
Image: www.chegg.com
Building Predictive Models
Once a regression model is built, we can use it to make predictions about future outcomes. By plugging in values for the independent variables, we can estimate the likely outcome of the dependent variable. This allows us to plan for future scenarios, optimize strategies, and make more informed decisions.
Real-World Applications
Multivariable methods are used across many fields. Here are a few examples:
- Finance: Predicting stock prices, assessing credit risk, and analyzing investment portfolios.
- Healthcare: Identifying risk factors for diseases, predicting patient outcomes, and personalizing treatment plans.
- Marketing: Optimizing advertising campaigns, identifying customer segmentation, and predicting customer behavior.
- Social Sciences: Understanding social phenomena, analyzing economic trends, and predicting voting behavior.
Navigating the Latest Trends and Developments
The field of multivariable analysis is constantly evolving. Advances in computing power and data availability have opened up new possibilities. Here’s a glimpse of some current trends:
- Machine Learning and Artificial Intelligence (AI): Deep learning algorithms are increasingly being integrated into multivariable analysis, enabling the identification of complex and non-linear relationships within data. This enhances predictive power and enables the discovery of hidden patterns.
- Big Data Analytics: Modern datasets are vast and often unstructured. Approaches like penalized regression and Bayesian methods are being developed to handle high-dimensional and sparse data, extracting valuable insights from immense datasets.
- Causal Inference: A growing focus on understanding causal relationships (rather than just correlations) is driving research into techniques like instrumental variables analysis and randomized controlled trials. These allow researchers to establish robust causal connections within complex systems.
Expert Tips for Effective Analysis
Applying multivariable methods effectively requires a combination of technical skills and critical thinking. Here are some expert tips:
- Start with a Clear Research Question: What are you trying to understand or predict? Define your objective before diving into data analysis.
- Ensure Data Quality: Garbage in, garbage out! Clean and prepare your data carefully to avoid errors and misleading results.
- Choose the Right Method: Consider the nature of your data and your research question when selecting the appropriate statistical technique.
- Interpret Results with Caution: Statistical significance does not always translate to practical relevance. Consider the context of your findings and the size of the effects.
- Don’t Overfit: Avoid creating models that are too complex and only fit the training data. Use techniques like cross-validation to ensure generalizability to new data.
Remember, multivariable analysis is a powerful tool, but it’s not a magic bullet. Always be mindful of the limitations of your data and assumptions, and interpret results with sound judgment. Critical thinking alongside statistical analysis is key for driving insightful and meaningful conclusions.
FAQs about Applied Regression Analysis and Multivariable Methods
Q: What is the difference between regression analysis and correlation analysis?
A: Correlation analysis measures the strength and direction of the relationship between two variables, but it doesn’t tell us if one variable influences the other. Regression analysis goes further by modeling the relationship, allowing us to predict the value of one variable based on the value of another (and potentially multiple others).
Q: Are multivariable methods complex to learn?
A: The fundamental concepts can be grasped with a good introductory understanding of statistics. However, mastering advanced techniques and interpreting complex results requires deeper knowledge and practice.
Q: How can I learn more about multivariable analysis?
A: There are many resources available, including online courses, textbooks, and workshops. Start with introductory materials and gradually explore more specialized topics as your knowledge grows.
Applied Regression Analysis And Other Multivariable Methods
Conclusion
Applied regression analysis and other multivariable methods offer a powerful toolkit for unlocking insights from complex data. By understanding the core concepts, choosing appropriate methods, and interpreting results with critical thinking, you can make informed decisions, identify hidden patterns, and drive progress in various fields.
Are you interested in learning more about applied regression analysis or other multivariable methods? Let us know in the comments below! We’re happy to discuss specific applications or answer any questions you may have.